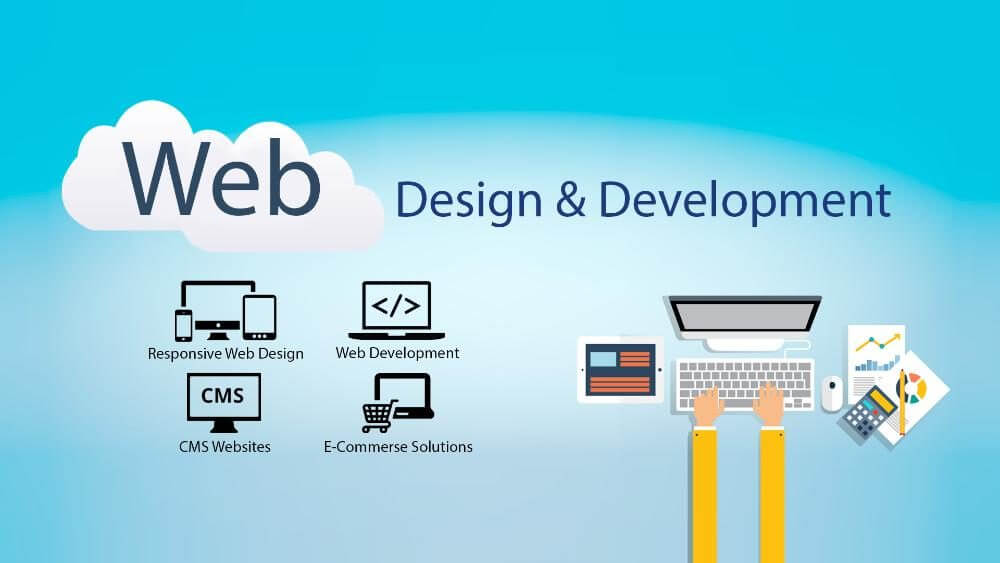
What’s Informative Websites?
Here are some major matrices that you can’t ignore the informative website. like Traffic-source, keyword stuffing, UI-UX Design, Informative pages of your
Here are some major matrices that you can’t ignore the informative website. like Traffic-source, keyword stuffing, UI-UX Design, Informative pages of your
The major limitation in defining AI as simply “building machines that are intelligent” is that it doesn’t actually explain what AI is and what makes a machine intelligent. AI is an interdisciplinary science with multiple approaches, but advancements in machine learning and deep learning are creating a paradigm shift in virtually every sector of the tech industry.
While a number of definitions of artificial intelligence (AI) have surfaced over the last few decades, John McCarthy offers the following definition in this 2004 paper (PDF, 106 KB) (link resides outside IBM), ” It is the science and engineering of making intelligent machines, especially intelligent computer programs. It is related to the similar task of using computers to understand human intelligence, but AI does not have to confine itself to methods that are biologically observable.”
However, decades before this definition, the birth of the artificial intelligence conversation was denoted by Alan Turing’s seminal work, “Computing Machinery and Intelligence” (PDF, 89.8 KB) (link resides outside of IBM), which was published in 1950. In this paper, Turing, often referred to as the “father of computer science”, asks the following question, “Can machines think?” From there, he offers a test, now famously known as the “Turing Test”, where a human interrogator would try to distinguish between a computer and human text response. While this test has undergone much scrutiny since its publish, it remains an important part of the history of AI as well as an ongoing concept within philosophy as it utilizes ideas around linguistics.
Stuart Russell and Peter Norvig then proceeded to publish, Artificial Intelligence: A Modern Approach (link resides outside IBM), becoming one of the leading textbooks in the study of AI. In it, they delve into four potential goals or definitions of AI, which differentiates computer systems on the basis of rationality and thinking vs. acting:
Human approach:
Ideal approach:
Accelerate data preparation and improve data governance with artificial intelligence
Pain Point: Analyses are only as good as the data that it contains, and as a result, many data scientists and analysts spend an exorbitant amount of time cleaning and organizing data to provide business value to their organizations. For example, raw data may not comply with your business taxonomy, and as a result, data may need to be classified in a specific way to provide context to stakeholders. Alternatively, you may not have a defined taxonomy for a new dataset yet; artificial intelligence can also help define clusters of data, segmenting groups based on shared characteristics. Either way, this can be a long, manual process for large datasets if left to an individual.
Solution: However, AI algorithms can assist in data classification tasks, reducing the cost and time to provide business insights. Labeled datasets can help categorize new data through supervised learning algorithms, while unsupervised learning can help businesses discover new clusters of data. We also asked businesses about this and we found that 39% of our survey participants identified data management and classification as a key use case of AI.